What Exactly Does It Mean to be a Systems Thinker?
Slapping a definition on the term and applying it to a real-life situation of modeling Support volume.
Happy Sunday, friends 🛬
This past week, I traveled to San Diego to jam on ideas with the Ness team. We’re remote-first so this rare, in-person time is so very crucial. We worked on ideas, planned out the next quarter, and shared plenty of delicious meals. Tons of laughing and fun sprinkled in for good measure (we’re hiring!).
Speaking of Ness, we have some exciting stuff on the horizon. Stay tuned!
This week’s dive is inspired by a recent podcast I’ve been digging - Between Two COO’s. If you’re into operations, I’d recommend subscribing. I’ll throw one more rec out - Supermanagers (fairly certain I’m late on this one). Start here with Scott Williamson, CPO at GitLab.
What are you listening to recently? Hit reply and let me know.
Hope you have a great week ahead!
Jeremey
Thanks for being a part of this newsletter. Every Sunday evening, I send a note about solving problems and wowing customers. Please say hi (reply here or at jeremeylduvall@gmail.com). I’d love to hear from you.
What is Systems Thinking anyway?
Last week, I listened to the latest episode of Between Two COO’s with Uplight COO Angela Tucci. Towards the end of the podcast, Angela offers a piece of advice for aspiring COOs:
Become a systems thinker.
I had three immediate thoughts:
“Yep - I’ve heard this advice before. Seems consistent!”
“Systems thinking feels obviously superior to the opposite…which is…well, I’m not sure. Unsystematic thinking? That can’t be right.”
“If pressed for a definition of systems thinking, I’m not exactly sure what I’d say. ‘Inputs something something outputs something else.’”
So, I dusted off my notes from Thinking in Systems with the aim of refreshing my memory on exactly what it means to be a systems thinker and why it’s so valuable. Then, I put together a practical example that highlights something I’m thinking about currently - modeling out our Support volume at Ness.
Starting with a rough definition
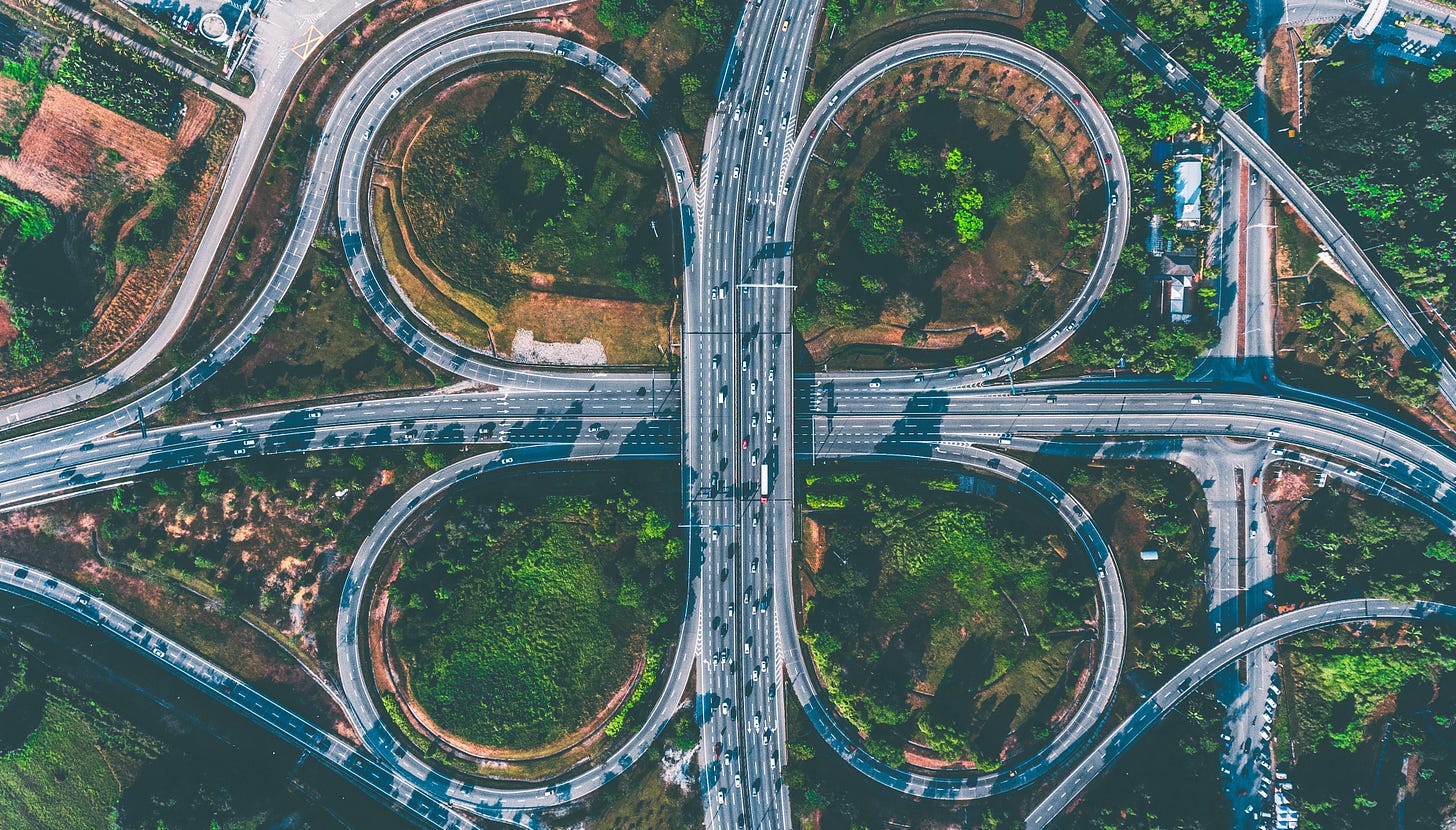
Pulling from my highlights, here’s a definition I cobbled together regarding a system:
A system is a set of individual elements that are interconnected in such a way that they achieve a specific outcome.
I’ve bolded the three distinct characteristics - individual elements, connections, and purpose.
Elements are often the most obvious piece of a system. If we take a simple example of a support queue, the elements could include incoming tickets, support tooling, and support agents sending replies.
“Interconnected” just means that elements within a system are connected in a way that:
Allows any element to impact the others
Means a system is more than just the sum of its parts.
Back to our support queue, customers submit tickets through a form. The tool routes those tickets to the appropriate agent who then responds. If a customer submits two tickets, they necessitate two replies from the agent. One element impacts another.
Now, the final piece - outcome or purpose. The most accurate way to define the purpose of a system is by watching it operate (credit to Donella Meadows!). As is often the case, behaviors speak much louder than stated goals.
A trip back to our support queue - let’s imagine our stated goal for customer support is to maintain a high CSAT score. However, we evaluate and drill into agents the importance of sending 50 replies per day to keep an empty queue. The actual purpose, then, is to keep the queue empty regardless of how we talk publicly about CSAT.
Why has system thinking become such a buzzword?
If systems thinking is all about complex interactions between many interdependent parts, the opposite then has to be linear thinking (hardly an original thought!).
Linear thinking involves a simple cause and effect between two variables that operate in predictable ways.
Think, “If A goes up, B will also go up by X%.”
Framed this way, it’s easier to see why systems thinking has become so valued. Very few situations have such a clear, simple cause and effect. There are always extraneous factors involved that generate unpredictable side effects.
A linear approach would assume that when incoming support volume increases by 20%, we’ll need 10 additional hours of agent time to clear the queue.
Anyone that’s worked in customer support understands that it’s rarely this cut and dry.
The linear approach fails to consider many ways the support system will naturally evolve as a result. For example, agents will probably begin responding faster after seeing more tickets flooding in. They might also develop a new suite of predefined replies further increasing RPH.
Compared to linear thinking, a systems perspective embraces complexity, understands how items are connected, and appreciates that situations are rarely simple.
Applying systems thinking to Support volume
Let’s take this systems thinking lens and apply it to a topic I’ve been thinking a lot about - support volume and SLAs. As we gear up for our first product launch at Ness, I’ve been tweaking a support model that anticipates incoming volume and forecasts hiring numbers, SLAs, etc with some decent level of accuracy. We’ll improve this over time as we get real numbers, but this gives us a starting point.
Side note: If you read Thinking in Systems (encouraged!) or dig deeper on the topic, you’ll start to read about terms like stock, stabilizing loops, feedback, etc. We’ll keep it simple though for now.
Our system includes incoming ticket volume and outgoing agent replies that culminate in a reply time SLA (service-level agreement). I’ll subject you to some rudimentary drawings to drive the point home.
A linear model would assume that almost everything is constant - incoming tickets are evenly spaced throughout the day, replies per hour are held constant, etc - making it very easy to predict what SLAs we could be striving for.
A systems approach understand that there are a variety of factors at play that will influence each piece of the chain. Some quick ones that come to mind include:
Incoming Volume
Contact rate and time distribution throughout the day
Percent of customers that self-serve
Distribution across channels (phone vs. email)
Product design and contact discoverability (how easy/hard it is to contact support)
Replies to resolution (which means each incoming ticket is essentially 2-3x tickets)
Outgoing Volume
Agent replies per hour
Internal tool efficiency
Training and agent setup
Agent experience
Scheduling vs. ticket volume (are agents waiting on tickets or do they have a queue?)
The point of drawing this complex model isn’t to get specific numbers in place for every factor; it’s to highlight the many levers you have at your disposal to impact the entire system.
Let’s imagine, for example, that your goal was to speed up your reply times. Even with just the quick list above, you have so many options. You could increase demands on your team, but you could also:
Improve the efficiency of internal tools so it’s easier for them to respond to tickets.
Change the intake form to automatically categorize requests and bucket them so agents can batch replies.
Tweak agent scheduling so everyone is dispersed throughout the day versus stepping on one another in the queue.
Wrapping it all up
Again, the purpose of systems thinking in my mind isn’t to list out every single factor that could influence a situation. Rather, it’s just to realize that the situation you’re facing is likely very complex.
Tweaking one thing will likely result in some unforeseen changes somewhere else. Stepping back and considering the whole system will give you a better understanding of how pieces work together and the variety of tools at your disposal.
That all adds up to more effective outcomes.